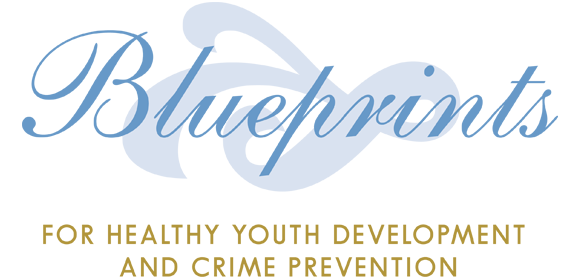
Growth Mindset for 9th Graders
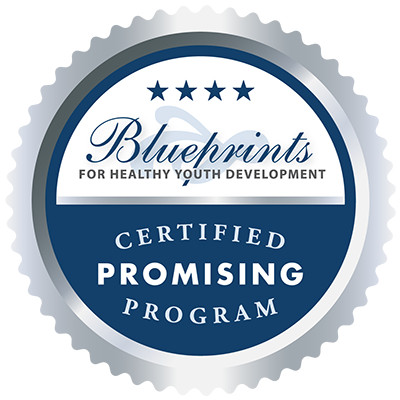
An online program for ninth-grade students as they transition to high school to improve grade point average and willingness to take difficult course work by changing the mindset toward learning.
Program Outcomes
- Academic Performance
Program Type
- Academic Services
- School - Individual Strategies
Program Setting
- School
Continuum of Intervention
- Universal Prevention
Age
- Late Adolescence (15-18) - High School
Gender
- Both
Race/Ethnicity
- All
Endorsements
Blueprints: Promising
Program Information Contact
PERTS (Project for Education Research That Scales)
Alejandra Zeiger
Email: support@perts.net
Website: https://perts.net/ninth-grade-mindset
For additional program and research information, see:
https://studentexperiencenetwork.org/national-mindset-study/
Program Developer/Owner
David Paunesku, Ph.D.
PERTS (Project for Education Research That Scales)
Brief Description of the Program
The program seeks to teach adolescents that intellectual abilities are not fixed but capable of growth with dedicated effort. It focuses on reducing negative beliefs about effort (e.g., having to try hard or ask for help means you lack ability), fixed-trait attributions (e.g., failure stems from low ability), and performance avoidance goals (e.g., never looking stupid). During regular school hours, students complete two self-administered online sessions that last approximately 25 minutes each and occur roughly 20 days apart. The first session starts in weeks 2-5 of the fall or spring semester, and the second session starts in weeks 5-10 of the same semesters. The sessions include scientific information but also ask students to help in communicating these ideas to others and to apply them to their own life. Since the intervention is computerized, materials can be delivered as designed without extensive researcher involvement or facilitator training and geographic constraints and logistical burdens are reduced, thereby providing scalable techniques to improve students' approach to learning and achievement in high school.
The program seeks to teach adolescents that intellectual abilities are not fixed but capable of growth with dedicated effort. It focuses on reducing negative beliefs about effort (e.g., having to try hard or ask for help means you lack ability), fixed-trait attributions (e.g., failure stems from low ability), and performance avoidance goals (e.g., never looking stupid). During regular school hours, students complete two self-administered online sessions that last approximately 25 minutes each and occur roughly 20 days apart. The first session starts in weeks 2-5 of the fall or spring semester, and the second session starts in weeks 5-10 of the same semesters. The sessions include scientific information but also ask students to help in communicating these ideas to others and to apply them to their own life.
The first session covers the basic idea of a growth mindset - that an individual's intellectual abilities can be developed by taking on challenging work, improving one's learning strategies, and asking for appropriate help. The second session invites students to deepen their understanding of the growth mindset and its application in their lives. Students are not told outright that they should work hard or employ particular study or learning strategies. Rather, effort and strategy revision are described as general behaviors through which students can develop their abilities and thereby achieve their goals.
Since the intervention is computerized, materials can be delivered as designed without extensive researcher involvement or facilitator training and geographic constraints and logistical burdens are reduced, thereby providing scalable techniques to improve students' approach to learning and achievement in high school.
Outcomes
Primary Evidence Base for Certification
Study 1 (Yeager et al., 2019; Zhu et al., 2019) found that, relative to the control group, the intervention group showed significantly higher
- GPA overall and in math/science classes
- Enrollment in advanced math classes
- Fixed-mindset beliefs.
Study 3
Yeager et al. (2016) found that, relative to the control group, the intervention group showed significantly
- Greater improvement in self-reported measures of a growth mindset
- Higher end-of-semester GPA for those with low prior achievement.
Brief Evaluation Methodology
Primary Evidence Base for Certification
Of the four studies Blueprints has reviewed, two studies (Studies 1 and 3) meet Blueprints evidentiary standards (specificity, evaluation quality, impact, dissemination readiness). The studies were done by the developer.
Study 1
Yeager et al. (2019) and Zhu et al. (2019) examined a nationally representative sample of ninth-grade students who were randomly assigned to an intervention group (N = 6,700) or a control group (N = 6,720). As designated in the pre-registration, Yeager et al. (2019) focused on 6,320 low-achieving students, while Zhu et al. (2019) focused on the full sample. The primary outcome was student GPA at the end of ninth grade, but fixed-mindset beliefs were measured at the end of the intervention sessions and enrollment in advanced math courses was measured in tenth grade.
Study 3
Yeager et al. (2016) used a randomized controlled trial to examine 3,676 ninth-grade students in 10 high schools. Students randomly assigned to the intervention and control groups completed posttest self-reports at the end of the program, and their grades in core courses were examined at the end of the semester.
Study 1
Yeager, D. S., Hanselman, P., Walton, G. M., Murray, J. S., Crosnoe, R., Muller, C., . . . Dweck, C. S. (2019). A national experiment reveals where a growth mindset improves achievement. Nature, 573, 364-369.
Zhu, P., Garcia, I., Boxer, K., Wadhera, S., & Alonzo, E. (2019). Using a growth mindset intervention to help ninth-graders: An independent evaluation of the National Study of Learning Mindsets. MDRC.
Study 3
Yeager, D. S., Romero, C., Paunesku, D., Hulleman, C., Schneider, B., Hinojosa, C., . . . Dweck, C. S. (2016). Using design thinking to improve psychological interventions: The case of the growth mindset during the transition to high school. Journal of Educational Psychology, 108(3), 374-391.
Subgroup Analysis Details
Subgroup differences in program effects by race, ethnicity, or gender (coded in binary terms as male/female) or program effects for a sample of a specific race, ethnic, or gender group.
- Study 1 (Yeager et al., 2019; Zhu et al,. 2019) tested for subgroup differences in program effects by race, and ethnicity and found equal benefits across subgroups. In addition, Zhu et al. (2019) tested for subgroup differences in program effects by gender and economic disadvantage (i.e., poverty status) and found equal benefits across subgroups.
- Study 3 (Yeager et al., 2016) did not test for subgroup effects defined by race, ethnicity, gender, sexual identity, economic disadvantage, geographic location, or birth origin.
Sample demographics including race, ethnicity, and gender for Blueprints-certified studies:
- The Study 1 sample was 49% female, 43% white, 24% Hispanic, 11% black, 4% Asian, and 18% multiple race/ethnicity.
- The Study 2 sample was 17% Hispanic/Latino, 6% Black/African American, 3% Native American/ American Indian, 48% White, non-Hispanic, 5% Asian/Asian American, and 21% from another or multiple racial groups. Forty-eight percent were female.
The Program Information Packet is intended for educators who are interested in learning about or implementing Growth Mindset for 9th Graders at their school. The information provided in this document help educators understand the research behind the program, the process for implementing it, and how its impact is tracked. The packet also provides guidance for introducing others to Growth Mindset for 9th Graders and a variety of helpful resources such as: a shareable brochure, a printable implementation checklist, and answers to the most frequently asked questions (accessible through an interactive Support Portal).
In addition to the Program Information Packet and the supporting resources it links to, there is a step-by-step guide within the Growth Mindset for 9th Graders platform. After creating an account and accessing a web-based Dashboard, educators will find interactive instructions that detail how to facilitate, launch, and monitor the program. The Dashboard also includes instructions on how to describe the program to students and suggestions on how to manage any potential technical issues.
Source: Washington State Institute for Public Policy
All benefit-cost ratios are the most recent estimates published by The Washington State Institute for Public Policy for Blueprint programs implemented in Washington State. These ratios are based on a) meta-analysis estimates of effect size and b) monetized benefits and calculated costs for programs as delivered in the State of Washington. Caution is recommended in applying these estimates of the benefit-cost ratio to any other state or local area. They are provided as an illustration of the benefit-cost ratio found in one specific state. When feasible, local costs and monetized benefits should be used to calculate expected local benefit-cost ratios. The formula for this calculation can be found on the WSIPP website.
Start-Up Costs
Initial Training and Technical Assistance
No information is available
Curriculum and Materials
Growth Mindset for 9th Graders is available for free to all high schools in the United States and can be accessed via the PERTS (Project for Education Research That Scales) website.
The Program Information Packet is intended for educators who are interested in learning about or implementing Growth Mindset for 9th Graders at their school. The information provided in this document helps educators understand the research behind the program, the process for implementing it, and how its impact is tracked. The packet also provides guidance for introducing others to Growth Mindset for 9th Graders and a variety of helpful resources such as: a shareable brochure, a printable implementation checklist, and answers to the most frequently asked questions (accessible through an interactive Support Portal).
In addition to the Program Information Packet and the supporting resources it links to, there is a step-by-step guide within the Growth Mindset for 9th Graders platform. After creating an account and accessing a web-based Dashboard, educators will find interactive instructions that detail how to facilitate, launch, and monitor the program. The Dashboard also includes instructions on how to describe the program to students and suggestions on how to manage any potential technical issues.
Licensing
No information is available
Other Start-Up Costs
Internet access is necessary to set up a Growth Mindset for 9th Graders account and to access the surveys. Students can participate in the program using either computers or on mobile devices. Students have the option to turn on the audio on the modules and have the content read aloud to them. For this reason, students should be provided with headphones as they complete both modules.
Intervention Implementation Costs
Ongoing Curriculum and Materials
No information is available
Staffing
The program is open for participation from June to May and can be implemented once per academic semester (once in the Fall and once in the Spring). Typically, educators implement the program during the Fall semester, as freshmen benefit from learning about growth mindset during this challenging and significant period of transition.
Educators and facilitators can decide in which subject areas they want to implement the program. Students should complete the two 30-minute online modules (a survey about growth mindset and a set of writing exercises) on their own during class, with modules completed about 1-4 weeks apart. Each individual student should only complete the program once. It typically takes about 2-4 weeks to finalize the program logistics for a school and less than one hour to register and get set up on the online platform.
Teachers and staff may want to dedicate an hour of (professional development) time to review and analyze the report data.
Other Implementation Costs
No information is available
Implementation Support and Fidelity Monitoring Costs
Ongoing Training and Technical Assistance
Technical assistance is available to answer specific questions about program implementation, and may be accessed via email or online.
Fidelity Monitoring and Evaluation
Each semester, PERTS generates a report (for schools with at least 30 participants) which shows the impact of the program on survey outcomes at your school and across other participating schools. This report can be downloaded directly from your Dashboard.
Ongoing License Fees
No information is available
Other Implementation Support and Fidelity Monitoring Costs
No information is available
Other Cost Considerations
No information is available
Year One Cost Example
No information is available
Program Developer/Owner
David Paunesku, Ph.D.Executive Director and Co-FounderPERTS (Project for Education Research That Scales)dave@perts.net perts.net
Program Outcomes
- Academic Performance
Program Specifics
Program Type
- Academic Services
- School - Individual Strategies
Program Setting
- School
Continuum of Intervention
- Universal Prevention
Program Goals
An online program for ninth-grade students as they transition to high school to improve grade point average and willingness to take difficult course work by changing the mindset toward learning.
Population Demographics
Ninth-grade students, particularly those with below-average school achievement.
Target Population
Age
- Late Adolescence (15-18) - High School
Gender
- Both
Race/Ethnicity
- All
Subgroup Analysis Details
Subgroup differences in program effects by race, ethnicity, or gender (coded in binary terms as male/female) or program effects for a sample of a specific race, ethnic, or gender group.
- Study 1 (Yeager et al., 2019; Zhu et al,. 2019) tested for subgroup differences in program effects by race, and ethnicity and found equal benefits across subgroups. In addition, Zhu et al. (2019) tested for subgroup differences in program effects by gender and economic disadvantage (i.e., poverty status) and found equal benefits across subgroups.
- Study 3 (Yeager et al., 2016) did not test for subgroup effects defined by race, ethnicity, gender, sexual identity, economic disadvantage, geographic location, or birth origin.
Sample demographics including race, ethnicity, and gender for Blueprints-certified studies:
- The Study 1 sample was 49% female, 43% white, 24% Hispanic, 11% black, 4% Asian, and 18% multiple race/ethnicity.
- The Study 2 sample was 17% Hispanic/Latino, 6% Black/African American, 3% Native American/ American Indian, 48% White, non-Hispanic, 5% Asian/Asian American, and 21% from another or multiple racial groups. Forty-eight percent were female.
Other Risk and Protective Factors
Improved attitudes and beliefs about learning (i.e., intellectual abilities are capable of growth) by reducing negative beliefs about effort, fixed-trait attributions and performance avoidance
Risk/Protective Factor Domain
- Individual
Risk/Protective Factors
Risk Factors
Protective Factors
*Risk/Protective Factor was significantly impacted by the program
Brief Description of the Program
The program seeks to teach adolescents that intellectual abilities are not fixed but capable of growth with dedicated effort. It focuses on reducing negative beliefs about effort (e.g., having to try hard or ask for help means you lack ability), fixed-trait attributions (e.g., failure stems from low ability), and performance avoidance goals (e.g., never looking stupid). During regular school hours, students complete two self-administered online sessions that last approximately 25 minutes each and occur roughly 20 days apart. The first session starts in weeks 2-5 of the fall or spring semester, and the second session starts in weeks 5-10 of the same semesters. The sessions include scientific information but also ask students to help in communicating these ideas to others and to apply them to their own life. Since the intervention is computerized, materials can be delivered as designed without extensive researcher involvement or facilitator training and geographic constraints and logistical burdens are reduced, thereby providing scalable techniques to improve students' approach to learning and achievement in high school.
Description of the Program
The program seeks to teach adolescents that intellectual abilities are not fixed but capable of growth with dedicated effort. It focuses on reducing negative beliefs about effort (e.g., having to try hard or ask for help means you lack ability), fixed-trait attributions (e.g., failure stems from low ability), and performance avoidance goals (e.g., never looking stupid). During regular school hours, students complete two self-administered online sessions that last approximately 25 minutes each and occur roughly 20 days apart. The first session starts in weeks 2-5 of the fall or spring semester, and the second session starts in weeks 5-10 of the same semesters. The sessions include scientific information but also ask students to help in communicating these ideas to others and to apply them to their own life.
The first session covers the basic idea of a growth mindset - that an individual's intellectual abilities can be developed by taking on challenging work, improving one's learning strategies, and asking for appropriate help. The second session invites students to deepen their understanding of the growth mindset and its application in their lives. Students are not told outright that they should work hard or employ particular study or learning strategies. Rather, effort and strategy revision are described as general behaviors through which students can develop their abilities and thereby achieve their goals.
Since the intervention is computerized, materials can be delivered as designed without extensive researcher involvement or facilitator training and geographic constraints and logistical burdens are reduced, thereby providing scalable techniques to improve students' approach to learning and achievement in high school.
Theoretical Rationale
Teaching adolescents that intellectual abilities are not fixed but capable of growth with dedicated effort can improve how they think or feel about themselves and how well they do in their schoolwork. Also, the defined user group to teach growth mindset is students making the transition to high school. Focusing on ninth-grade students and improving the transition to high school is an important policy objective, as students who do not successfully complete ninth-grade core courses have a dramatically lower rate of high school graduation and much poorer life prospects.
Theoretical Orientation
- Skill Oriented
Brief Evaluation Methodology
Primary Evidence Base for Certification
Of the four studies Blueprints has reviewed, two studies (Studies 1 and 3) meet Blueprints evidentiary standards (specificity, evaluation quality, impact, dissemination readiness). The studies were done by the developer.
Study 1
Yeager et al. (2019) and Zhu et al. (2019) examined a nationally representative sample of ninth-grade students who were randomly assigned to an intervention group (N = 6,700) or a control group (N = 6,720). As designated in the pre-registration, Yeager et al. (2019) focused on 6,320 low-achieving students, while Zhu et al. (2019) focused on the full sample. The primary outcome was student GPA at the end of ninth grade, but fixed-mindset beliefs were measured at the end of the intervention sessions and enrollment in advanced math courses was measured in tenth grade.
Study 3
Yeager et al. (2016) used a randomized controlled trial to examine 3,676 ninth-grade students in 10 high schools. Students randomly assigned to the intervention and control groups completed posttest self-reports at the end of the program, and their grades in core courses were examined at the end of the semester.
Outcomes (Brief, over all studies)
Primary Evidence Base for Certification
Study 1
Yeager et al. (2019) and Zhu et al. (2019) found that the intervention group had significantly higher overall GPA and math/science GPA than the control group. The intervention effects appeared for both the full sample and the subsample of low-achieving students. The intervention also improved a measure of fixed-mindset beliefs and, for the sample of all students, enrollment in tenth-grade advanced math classes.
Study 3
Yeager et al. (2016) found that the intervention group showed significantly greater improvement in self-reported measures of a growth mindset than the control group. For the end-of-semester GPA, the intervention group also did significantly better than the control group but only for those with low prior achievement. For a related measure of poor grades, the intervention group did significantly better than the control group overall and for those with low prior achievement.
Outcomes
Primary Evidence Base for Certification
Study 1 (Yeager et al., 2019; Zhu et al., 2019) found that, relative to the control group, the intervention group showed significantly higher
- GPA overall and in math/science classes
- Enrollment in advanced math classes
- Fixed-mindset beliefs.
Study 3
Yeager et al. (2016) found that, relative to the control group, the intervention group showed significantly
- Greater improvement in self-reported measures of a growth mindset
- Higher end-of-semester GPA for those with low prior achievement.
Effect Size
In Study 1 (Yeager et al., 2019), the GPA effect size of .11 for the low-achieving subsample was small by the usual standards, but the authors argued that for GPA, an effect of that size was substantively important. The GPA effect size of .04 for the full sample in Study 1 (Zhu et al., 2019, Appendix Table C.1) was even smaller.
Generalizability
Two studies meet Blueprints standards for high-quality methods with strong evidence of program impact (i.e., "certified" by Blueprints): Study 1 (Yeager et al., 2019; Zhu et al., 2019) and Study 3 (Yeager et al., 2016). The samples for both studies included ninth-grade students.
Study 1 examined students from a nationally representative sample of U.S. high schools and compared the treatment group to an active control group.
Study 3 examined students came from a national convenience sample of public high schools in California, New York, Texas, Virginia, and North Carolina and compared the treatment group to an active control group.
Potential Limitations
Additional Studies (not certified by Blueprints)
Study 2 (Yeager et al., 2016)
- No behavioral outcome measures
- Unclear on use of baseline outcomes as covariates
- Posttest effects only for risk and protective factors
Yeager, D. S., Romero, C., Paunesku, D., Hulleman, C., Schneider, B., Hinojosa, C., . . . Dweck, C. S. (2016). Using design thinking to improve psychological interventions: The case of the growth mindset during the transition to high school. Journal of Educational Psychology, 108(3), 374-391.
Study 4 (Paunesku et al., 2015)
- Details on attrition unclear
- Incomplete tests for baseline equivalence
- No tests for differential attrition (and only the sample size for the analysis sample was reported)
- No main effects for the primary outcome (GPA), with condition differences only for the at-risk sample
Paunesku, D., Walton, G. M., Romero, C., Smith, E. N., Yeager, D. S., & Dweck, C. S. (2015). Mind-set interventions are a scalable treatment for academic underachievement. Psychological Science, 26, 784 -793. http://dx.doi.org/10.1177/0956797615571017
Endorsements
Blueprints: Promising
Program Information Contact
PERTS (Project for Education Research That Scales)
Alejandra Zeiger
Email: support@perts.net
Website: https://perts.net/ninth-grade-mindset
For additional program and research information, see:
https://studentexperiencenetwork.org/national-mindset-study/
References
Study 1
Certified
Yeager, D. S., Hanselman, P., Walton, G. M., Murray, J. S., Crosnoe, R., Muller, C., . . . Dweck, C. S. (2019). A national experiment reveals where a growth mindset improves achievement. Nature, 573, 364-369.
Certified
Zhu, P., Garcia, I., Boxer, K., Wadhera, S., & Alonzo, E. (2019). Using a growth mindset intervention to help ninth-graders: An independent evaluation of the National Study of Learning Mindsets. MDRC.
Study 2
Yeager, D. S., Romero, C., Paunesku, D., Hulleman, C., Schneider, B., Hinojosa, C., . . . Dweck, C. S. (2016). Using design thinking to improve psychological interventions: The case of the growth mindset during the transition to high school. Journal of Educational Psychology, 108(3), 374-391.
Study 3
Certified
Yeager, D. S., Romero, C., Paunesku, D., Hulleman, C., Schneider, B., Hinojosa, C., . . . Dweck, C. S. (2016). Using design thinking to improve psychological interventions: The case of the growth mindset during the transition to high school. Journal of Educational Psychology, 108(3), 374-391.
Study 4
Paunesku, D., Walton, G. M., Romero, C., Smith, E. N., Yeager, D. S., & Dweck, C. S. (2015). Mind-set interventions are a scalable treatment for academic underachievement. Psychological Science, 26, 784 -793. http://dx.doi.org/10.1177/0956797615571017
Study 1
The two articles used the same data but focused on different samples and were done independently. Also, Yeager et al. (2019) followed the methods laid out in the pre-registration more strictly than Zhu et al. (2019).
Summary
Yeager et al. (2019) and Zhu et al. (2019) examined a nationally representative sample of ninth-grade students who were randomly assigned to an intervention group (N = 6,700) or a control group (N = 6,720). As designated in the pre-registration, Yeager et al. (2019) focused on 6,320 low-achieving students, while Zhu et al. (2019) focused on the full sample. The primary outcome was student GPA at the end of ninth grade, but fixed-mindset beliefs were measured at the end of the intervention sessions and enrollment in advanced math courses was measured in tenth grade.
Yeager et al. (2019) and Zhu et al. (2019) found that, relative to the control group, the intervention group showed significantly higher
- GPA overall and in math/science classes
- Enrollment in advanced math classes
- Fixed-mindset beliefs.
Evaluation Methodology
Design:
Recruitment: The study began with the population of ninth-grade students in all regular public U.S. schools in 2015-2016. A professional research company recruited a stratified random sample of 139 schools, 76 agreed to participate, and 65 provided data. A generalizability index and standardized mean differences (Yeager et al., 2019, supplement section 3) demonstrated that the 65 schools were generalizable to the population. A total of 13,420 students in the schools received parental consent and participated in the study. However, in accord with the pre-registration, Yeager et al. (2019) mostly examined a subsample of lower-achieving students (N = 6,320) who had a GPA at or below their school median.
Zhu et al. (2019) excluded two schools because of problems with the reported data, leaving a sample of 63 high schools and 11,888 ninth-grade students. The sample still generalized to the population of regular U.S. public schools (Appendix Table B.1).
Assignment: Students were randomly assigned within schools to the intervention (N = 6,700) and control conditions (N = 6,720) when they first signed into the study website. Both conditions used an online program. The intervention group read articles and completed lessons on a growth mindset, while the control group read articles and completed lessons on brain science.
Zhu et al. (2019) limited the sample to students with nonmissing GPA scores at the end of ninth grade. Among these students, 5,916 were randomly assigned to the program group, while 5,972 were randomly assigned to the control group
Assessments/Attrition: The key outcomes were assessed at the end of ninth grade. With schools implementing the program early in either the fall or spring semester, the assessment came 3-7 months after the end of the program. Other measures came from a survey completed immediately after the program end and from enrollment data for tenth grade. At the end of ninth grade, attrition in Yeager et al. (2019) ranged, depending on the outcome, from 6.6-13.3% for the control group and from 7.2-13.3% for the intervention group (Table 6.8.1). For the tenth-grade measures, however, attrition was much higher, with data available for 41 of 65 schools (63%) and 6,690 of 13,420 students (50%). Attrition in Zhu et al. (2019) ranged from 11-12%.
Sample:
The sample was 49% female, 43% white, 24% Hispanic, 11% black, 4% Asian, and 18% multiple race/ethnicity (supplement Table 6.2.1). About 29% had mothers with a bachelor's degree or higher.
Measures:
The primary pre-registered outcome was the grade point average (GPA) in core ninth-grade classes (mathematics, science, English or language arts, and social studies), which came from administrative data sources of the schools. A related binary measure distinguished those with a D/F GPA below 2.0 (or below 1.0 in Zhu et al., 2019). Yeager et al. (2019) also focused on the math/science GPA, while Zhu et al. (2019) focused on the math GPA. Teachers, researchers, and the private companies that collected the data were kept blinded to student assignment.
An exploratory, non-registered measure used for all students equaled the rate of taking an advanced mathematics course (algebra II or higher) in tenth grade, the year after the intervention.
One measure came from the posttest survey. The self-reported fixed-mindset scale measured agreement with three statements such as "You have a certain amount of intelligence, and you really can't do much to change it."
Two other measures served as moderators (both identified in the pre-registration). The school achievement level was based on publicly available indicators of school resources and school performance on state and national exams. The growth-mindset or challenge-seeking norms of each school was based on the average number of challenging mathematical problems chosen by control students at the end of their sessions. Supplement section 10 of Yeager et al. (2019) provides evidence of the validity of the measure.
Analysis:
The analyses in Yeager et al. (2019) were carried out as specified in the pre-registration plan, and Zhu et al. (2019) used similar models. The main effects analyses used cluster-robust school-fixed-effects regressions, while the heterogeneity or moderation analyses used "hybrid" mixed-effects models with school fixed intercepts and random slopes. The models controlled for baseline outcomes and numerous covariates. Most models used weights to represent the population of regular public high schools in the U.S. To check for the robustness of the results in Yeager et al. (2019), analysts who were blinded to the study hypotheses and the identities of the variables conducted Bayesian, machine-learning robustness tests.
Intent-to-Treat: The analyses included all randomized students with data, regardless if they completed the sessions. Yeager et al. (2019) imputed missing baseline data, while Zhu et al. (2019) did not. A complier average causal effects analysis in Yeager et al. (2019) used randomization as an instrumental variable for students completing the intervention; it yielded the same conclusions as the ITT analysis but had slightly larger effect sizes (see supplement section 9).
Outcomes
Implementation Fidelity:
In the median school, treated students viewed 97% of screens and wrote a response for 96% of open-ended questions. Supplement section 5.6 presents five measures of fidelity such as the proportion completing open-ended responses and self-reported avoidance of distraction. The means for both conditions ranged from 0.888 to 0.950, indicating high fidelity.
Baseline Equivalence:
In supplement Table 6.7.1 of Yeager et al. (2019), tests for eight baseline measures (gender, mother with a college degree, black, Asian, Hispanic, white, fixed mindset scale, and GPA) showed no significant differences for the randomized sample (p < .05), and all standardized mean differences fell below 0.04.
Zhu et al. (2019) tested 15 baseline measures for the analysis sample. They found two significant differences, in favor of the control group. Both the average GPA and math GPA were higher in the control group, but the standardized differences were only 0.05 (Appendix Table B.2). As a check, dropping the four to eight schools that most differed on GPA did little to change the program effects (Appendix Table B.3).
Differential Attrition:
Yeager et al. (2019) examined the issue in most detail. In supplement Table 6.9.1, all eight baseline measures (gender, mother with a college degree, black, Asian, Hispanic, white, fixed mindset scale, and GPA) differed for completers and dropouts, with effect sizes as large as -.934 (for GPA, the key outcome). However, the predictors of attrition appeared similar across conditions. In supplement Table 6.8.1, tests for five baseline outcomes (core GPA, fixed mindset scale, growth mindset indicator, number of hard problems selected, and hypothetical challenge-seeking) showed no significant differences between the rate of treatment attrition and the rate of control attrition. Further, supplement Tables 6.10.1 and 6.10.2 show baseline equivalence on the eight baseline measures reported in Table 6.9.1 for the analysis sample of students who were not missing data. For eight baseline measures, none of the condition differences reached significance, and the largest effect size (GPA) was only -.032.
For Zhu et al. (2019), the tests for baseline equivalence in the analysis sample offered additional evidence of the similarity across conditions in the predictors of attrition.
Posttest:
Low-achieving subsample. For this subsample (Yeager et al., 2019), intervention students had higher GPAs in core classes at the end of the ninth grade than control students. The authors argued that the effect size of .11 was substantial given the subsample and difficulty in raising GPA. The effect was replicated with robustness tests using the Bayesian machine-learning algorithm. Significant or near significant intervention effects further emerged for GPA in mathematics (p = .074) and science (p < .001) and for a binary measure of a D/F GPA under 2.0. Also, for this subsample and relative to the control condition, intervention students showed significantly fewer fixed mindset beliefs at posttest (effect size = 0.33).
Full sample. Although pre-specified to be small, intervention effects on GPA for the full sample in Yeager et al. (2019, Extended Data Table 1) proved to be significant.
Zhu et al. (2019) found significantly higher GPAs and math GPAs and significantly fewer GPAs below 1.0 for the intervention group than the control group. They also reported that the intervention group agreed significantly less often with five of six items on fixed mindset attitudes and beliefs, was significantly more likely to say they would choose hard math problems and, when given the opportunity, chose significantly more difficult problems for a worksheet.
Moderation: The two moderation tests in Yeager et al. (2019) were specified in the pre-registration and began by demonstrating that intervention effects varied significantly across schools. For the lower-achieving subsample, the intervention had significantly stronger effects on overall GPA and math/science GPA in schools with 1) lower achievement levels and 2) behavioral norms that supported a growth mindset or challenge-seeking peer norms. These results were confirmed with robustness tests using the Bayesian machine-learning algorithm.
For the full sample in Zhu et al. (2019), the program impact varied by one student characteristic. The program impacted academic performance for lower-performing students, but not for higher-performing ones. The program impact also varied by two school characteristics. First, the impact was strongest in schools in the middle range of prior achievement (which differed somewhat from the findings of Yeager et al., 2019). Second, the impact was strongest in schools with high prevalence of a growth mindset and student challenge-seeking behavior (which replicated the findings of Yeager et al., 2019).
Long-Term:
Non-registered outcome: Analysis of taking advanced mathematics courses in tenth grade in Yeager et al. (2019) began with the full sample of both higher- and lower-achieving students, but only 41 of the 65 schools provided the data. The results showed that the intervention group had a significantly greater likelihood of taking the advanced courses than the control group. This effect was significantly stronger in the highest-achieving schools, a finding in the opposite direction of the moderation for GPA and suggestive of the benefits of the program for high-achieving schools.
Study 2
The article reported as Study 2 and Study 3 consisted of two parts, each with a different sample and design. The parts differed enough to be presented separately. The first (called Study 1 in the article but treated here as Study 2 in the Blueprints writeup) compared the revised Growth Mindset program to the original program, while the second (called Study 2 in the article but treated here as Study 3 in the Blueprints writeup) compared the revised Growth Mindset program to a control group.
Summary
Yeager et al. (2016) used a randomized controlled trial to examine 7,501 ninth-grade students in 69 high schools. Students randomly assigned to receive the revised version of the program or the original version completed posttest self-reports on measures of a fixed mindset.
Yeager et al. (2016) found that the intervention group did significantly better than the control group in reducing
- Self-reported measures of a fixed-mindset.
Recruitment: The study recruited 69 high schools in the United States and Canada via advertisements, social media, and presentations to school districts. A total of 7,501 ninth-grade students (predominately ages 14-15) provided data during the winter of 2015. About 40% of the students in the schools participated.
Assignment: Random assignment by a web server occurred during the initial online session. The intervention group (N = 3,665 for the analysis sample) received the revised version of the program, while the control group (N = 3,480 for the analysis sample) received the "original" version of the program.
Assessments/Attrition: Baseline data came during the initial (Time 1) online session, with the intervention following immediately afterward. The posttest (Time 2) session followed one to four weeks later. It first involved a second round of content and then completion of the outcome measures. The study did not mention attrition, but the analysis sample of 7,145 listed in Table 3 represented 95% of the total of 7,501 students who began the Time 2 session.
Sample:
Participants were diverse: 17% Hispanic/Latino, 6% Black/African American, 3% Native American/ American Indian, 48% White, non-Hispanic, 5% Asian/Asian American, and 21% from another or multiple racial groups. Forty-eight percent were female, and 53% reported that their mothers had earned a bachelor's degree or greater.
Measures:
All measures came from student self-reports.
- Fixed mindset. Three items asked for agreement with statements about the inability to change intelligence and being a math person (alpha = .74).
- Challenge-seeking: The "Make-a-Math-Worksheet" Task. When asked to create their own math worksheet, students could select easy or challenging problems. The measure equaled the number of easy problems minus the number of hard problems selected.
- Challenge-seeking: Hypothetical scenario. Students were asked if they would choose an easy or hard math assignment; high values indicated selection of easy problems and corresponded to the avoidance of challenge.
- Fixed-trait attributions. Students rated how likely they were after receiving a bad grade on an important math assignment to think that 1) they were not very smart at math or 2) they could get a better grade by studying more. The two items were averaged.
- Performance avoidance goals. Students rated their agreement with the statement, "One of my main goals for the rest of the school year is to avoid looking stupid in my classes."
Table 2 presents some correlations among these measures and others related to grit and self-control that offered evidence of measurement validity.
Analysis: The text refers (p. 382) to checks for violations of the linear model but otherwise gave no details on the analysis. Table 3 used t-tests for condition means differences, mostly for the posttest measures and once for a change score. It is unclear if the means were adjusted for covariates.
Intent-to-Treat: The authors noted that they obtained data from students even if they didn't finish the intervention session.
Outcomes
Implementation Fidelity: The online version delivered the program identically to all participants. In addition, a measure of the extent of distraction during the sessions was low for both conditions, and the intervention group reported higher scores than the control group on their interest in the program and their feeling that they learned something.
Baseline Equivalence: The authors reported no significant differences between conditions in terms of demographics (gender, race, ethnicity, special education, parental education), prior achievement, or fixed mindset.
Differential Attrition: Not examined, as attrition was likely low.
Posttest:
The key results in Table 3 demonstrated that the intervention group did better on all five outcomes: greater reduction of a fixed mindset, fewer easy worksheet problems chosen (d = .19), selection of an easy homework assignment (d = .19), fewer fixed-trait attributions (d = .07), and lower performance-avoidance goals (d = .06). Tests for moderation indicated that, for some of the outcomes, the program most benefited students with a low baseline fixed-mindset or with high prior achievement.
Long-Term:
Not examined.
Study 3
The article reported as Study 2 and Study 3 consisted of two parts, each with a different sample and design. The parts differed enough to be presented separately. The first (called Study 1 in the article but treated here as Study 2 in the Blueprints writeup) compared the revised Growth Mindset program to the original program, while the second (called Study 2 in the article but treated here as Study 3 in the Blueprints writeup) compared the revised Growth Mindset program to a control group.
Summary
Yeager et al. (2016) used a randomized controlled trial to examine 3,676 ninth-grade students in 10 high schools. Students randomly assigned to the intervention and control groups completed posttest self-reports at the end of the program, and their grades in core courses were examined at the end of the semester.
Yeager et al. (2016) found that, relative to the control group, the intervention group showed significantly
- Greater improvement in self-reported measures of a growth mindset
- Higher end-of-semester GPA for those with low prior achievement.
Evaluation Methodology
Design:
Recruitment: Students came from a national convenience sample of 10 public high schools in California, New York, Texas, Virginia, and North Carolina. One additional school was recruited, but validated student achievement records could not be obtained. The schools had ninth-grade enrollment between 100 and 600 students, moderate poverty indicators (e.g., free or reduced-price lunch), and moderate representation of students of color. The student sample included 3,676 ninth graders.
Assignment: Students were randomly assigned to the intervention group or control group by software during the first online session. For the analysis sample, the intervention group had 1,646 students and the control group had 1,630 students. Designed to parallel the intervention activities, the control activities provided information about the transition to high school, included exercises to retain the material, and presented images to make the material interesting.
Assessments/Attrition: During the first online session, both conditions completed baseline measures and then the first part of the program. The time 2 posttest session came 1 to 4 weeks after the time 1 baseline session, and school grades came at the end of the semester (at least a few weeks after the time 2 session but sometimes longer).
At baseline, 96% of eligible students participated. At posttest, 183 students (5%) did not enter their names accurately and were not matched to the baseline session, and an additional 291 students (8%) completed the baseline but not the posttest session. The analysis sample listed in Tables 3, 5, and 6 ranged from 89-94% of the randomized sample. Attrition would also include one school that did not provide any data, but the study provided no figures on the losses from this school.
Sample: Student participants were 29% Hispanic/Latino, 17% Black/African American, 3% Native American/American Indian, 30% White, non-Hispanic, 6% Asian/Asian American, and 15% from another or multiple racial groups. Forty-eight percent were female, and 52% reported that their mothers had earned a bachelor's degree or greater.
Measures:
Data came from student self-reports and school records gathered by an independent research agency. The two primary preregistered outcomes included:
- Ninth grade GPA. The measure averaged letter grades for science, math, and English.
- Poor grade performance. A dichotomous measure indicated an average GPA of D+ or below.
Four additional measures of hypothetical willingness to select easy math problems, a fixed mindset, fixed-trait attributions, and performance-avoidance goals were measured identically as in Study 2.
Analysis: Analyses of the two primary outcomes used regression models with controls for five covariates, baseline mindset, and prior achievement (an average of eighth-grade GPA and test scores). Fixed effects for school did not change the results.
Intent-to-Treat: The analyses used all available data. For schools, one was lost because of not providing data. For students, all who began the baseline session were retained, regardless of participation or completion of the posttest session.
Outcomes
Implementation Fidelity: During the time 1 baseline session, both intervention and control students saw an average of 96% of the screens. Among those who completed the time 2 posttest session, intervention students saw 99% of screens, compared with 97% for control students. The intervention students rated their programs as significantly more interesting than the control students.
Baseline Equivalence: The authors reported no significant differences between conditions in terms of demographics (gender, race, ethnicity, special education, parental education), prior achievement (of both schools and individuals), or fixed mindset.
Differential Attrition: The authors noted only that the students completing time 1 baseline materials but not time 2 posttest materials did not vary by condition.
Posttest:
Table 3 shows significant effects for all four risk and protection outcomes: a fixed mindset, fixed-trait attributions (d = .13), performance-avoidance goals (d = .11), and willingness to select easy problems. There was some evidence in moderation tests that high achieving students most improved on these outcomes.
For GPA, as justified by the pre-registered hypotheses, the results reported only moderated effects, not main effects. The intervention times prior achievement interaction effect was significant such that the intervention most benefited students with low prior achievement (d = .10 and p < .05 at one standard deviation below the mean). The intervention effect was near zero for students with high prior achievement.
For poor grade performance, there was a significant main effect of the program (d = .10), a significant effect for one standard deviation below the mean of prior achievement (d = .13), and a non-significant effect at one standard deviation above the mean.
Long-Term: Not examined.
Study 4
Study 4 (Paunesku et al., 2015) was the pilot test for Studies 2 and 3 (Yeager et al., 2016) and evaluated two different mind-set interventions - one for growth mind-set of intelligence and a second for sense of purpose - as compared to a control group. As Paunesku et al. (2015) explain on p. 785: Academic-mind-set interventions target students' core beliefs about school and learning, such as "Can I learn and grow my intelligence?" (growth-mind-set beliefs) and "Why should I learn?" (sense-of-purpose beliefs). Growth-mind-set interventions convey that intelligence can grow when students work hard on challenging tasks - and thus that struggle is an opportunity for growth, not a sign that a student is incapable of learning. Sense-of-purpose interventions encourage students to reflect on how working hard and learning in school can help them accomplish meaningful goals beyond the self, such as contributing to their community or being examples for other people, which help students relate course content to their lives. However, they are framed more broadly, in terms of the value of school in general and aim to sustain students' motivation when schoolwork is boring or frustrating but foundational to learning.
Summary
Paunesku et al. (2015) randomly assigned 1,594 students (the analytic sample) to a control condition or to one of three intervention conditions: 1) growth-mind-set intervention, 2) sense-of-purpose intervention, or 3) the two interventions combined. The primary outcome was GPA at the end of the intervention semester.
Paunesku et al. (2015) found that at the posttest, compared to the control group, participants in the growth mind-set intervention earned significantly higher:
- GPAs (but only for at-risk students)
- Self-reported measures of growth mindset (a risk and protective factor)
- Self-reported measures of meaningfulness of schoolwork (a risk and protective factor).
Evaluation Methodology
Design:
Recruitment: Thirteen high schools located in the eastern, western, and southwestern United States were recruited via presentations by researchers to educators or brief phone meetings. Participating high schools agreed to try and enroll 100 or more students, to provide academic outcomes, and to select a coordinator to recruit teachers and to ask teachers to create accounts on the study web site (http://www.perts.net/). The number of teachers and students recruited for the study was not reported.
Assignment: After signing into the study web site, each student was individually randomly assigned to a control condition or to one of three intervention conditions: growth-mind-set intervention, sense-of-purpose intervention, or the two interventions combined. For all groups, the growth-mind-set intervention (or related control materials) was delivered in two sessions. Both sessions were administered in the school computer lab during the spring semester, between January and May 2012. The sample size for the randomized sample was not reported.
Assessments/Attrition: The outcome was measured at the end of the spring semester. With the intervention occurring from January to May, the measurement served as a posttest. The analytic sample focused on 1,594 students for whom complete data were available.
Sample: Twelve of the high schools were public (one was private); of the public schools, 4 were charter schools. The sample was evenly divided in terms of gender. One-third of students were Hispanic, just under one-quarter (23%) were White, 17% were Asian, 11% were Black and 15% identified as "other" or mixed race. The majority (82%) of the sample was in 9th grade. Four percent were in 10th grade, 10% were in 11th grade and 4% were in 12th grade. Income data was not collected at the student level, but the participating schools varied widely in socioeconomic characteristics: In five schools, almost no students received free or reduced lunch because of low household income; in six, more than half of students did.
Measures:
Psychological measures
- Growth mindset: Authors measured growth mindset using two items: "You can learn new things, but you can't really change your basic intelligence" and "You have a certain amount of intelligence and you really can't do much to change it." (α = .84).
- Meaningfulness-of-schoolwork: In addition, students' construal of mundane academic tasks was measured using a meaningfulness-of-schoolwork task, which assesses whether students view schoolwork (e.g., "Doing your math homework") at a low, mechanical level (e.g., "Typing numbers into a calculator and writing formulas") or at a high level relevant to learning and growth ("Building your problem-solving skills"). Eight items formed a reliable composite (α = .72).
Academic measures - Schools provided participating students' transcripts. The authors calculated each student's end-of-semester GPA in core academic courses (i.e., math, English, science, and social studies) in the fall (preintervention) and in the spring (postintervention) as follows:
- GPA: Ten schools coded students' performance on a five-letter grading scale (A, B, C, D, F), whereas three assigned "no credit" (NC) in place of Ds and Fs. To numerically transform letter grades for analysis, the authors always coded A, B, and C as 4, 3, and 2, respectively. Because there was no single a priori basis by which to code D, F, and NC, the authors presented results using the following coding scheme: F = 0, D = 1, and NC = 1 (because D and NC are immediately below C).
Analysis: For the psychological outcomes, authors conducted a linear regression analysis that controlled for pre-study psychology measures (e.g., pre-study beliefs about intelligence were controlled for in the analysis measuring growth mindset outcomes; preintervention meaningfulness of schoolwork was controlled for in the schoolwork models). For the core GPA outcome measured the semester after the intervention, the authors conducted a linear regression analysis that included risk of dropping out from high school using thresholds created by the Consortium for Chicago School Research from official records collected by Chicago public schools (0 = not at risk, 1 = at risk), a dummy variable for each intervention condition, and a dummy variable for each Risk × Intervention (growth mind-set, sense of purpose, combined) interaction. Covariates included pre-study GPA, race, gender, and school.
Intent-to-Treat: The authors reported following an intent-to-treat protocol by including students who completed the first session even if they did not complete the second session. Only those without grade-point data were excluded.
Outcomes
Implementation Fidelity: Not reported.
Baseline Equivalence: Not tested for the psychological measures or demographic characteristics. The authors report: "In the semester before the intervention, the intervention and control groups did not differ in GPA, ts < 1" (p. 788).
Differential Attrition: Not tested and since the sample size for the randomized sample was not reported, it is not clear whether attrition was high.
Posttest: The study found no significant main effects for GPA. Compared to the control group, participants in the growth mind-set intervention earned significantly higher GPAs at the posttest (b = 0.13) but only for at-risk students. Similarly, compared to the control group, students in the sense-of purpose intervention earned significantly higher grades (b = 0.17), again only for at-risk students. There was no effect (overall or subgroup) on GPA for the combined intervention.
In terms of risk and protective factors, at the posttest, students in the growth mind-set intervention showed significantly higher posttest scores on the growth mindset outcomes than students in the control group (β = 0.17). There was no impact on growth mindset outcomes for the sense-of-purpose intervention (compared to the control group) or the combined interventions (compared to control students). In addition, the growth mind-set treatment group showed significantly higher posttest scores on the meaningfulness of schoolwork outcomes compared to the control group (β = 0.11), as did the sense-of-purpose treatment group compared to control students (β = 0.17). There was no impact for the combined interventions when compared to the control group.
Long-Term: Not tested.